Henry is in his second year as a PhD candidate in the David A. Dunlap Department of Astronomy and Astrophysics at the University of Toronto, specializing in using deep learning to further the understanding of our Milky Way Galaxy.
He completed an honours BSc majoring in physics and astronomy and MSc in astronomy and astrophysics from the University of Toronto before beginning his PhD.
When he isn’t doing research, you can find him wishing for clear skies to do some amateur astrophotography.
How did you first become interested in Astronomy and Astrophysics?
I was raised in Hong Kong where it has one of the worst light pollutions in the world so you can hardly see any stars. My first exposure to astronomy was the Mars opposition in 2003 – when Mars is at its closest distance to Earth in almost 60,000 years. This experience made me interested in astronomy. Along the way growing up, I have a few good mentors and friends to keep me hooked up in this field.
Can you tell us a little bit about your specific field of research?
My work mostly focused on the field of galactic dynamics and machine learning. Even thought we are living inside the disc of the Milky Way Galaxy, there are still so much we do not know about it even thought it is the only galaxy we can study in detail. My current research focuses on using new but robust machine learning methods like deep learning to analyze big astronomical survey datasets. Deep learning refers to the use of multi-layer artificial neural network to let computers learn the relation between input and output of data. We apply deep neural network on large dataset such as spectroscopic data from the SDSS’s Apache Point Observatory Galaxy Evolution Experiment (APOGEE) and ESA’s Gaia astrometric observations to help us better understand the Milky Way Galaxy like its current dynamics and formation history.
What’s the most exciting thing about your research?
The most exciting thing is I always get to learn the state-of-the-art research in deep learning such as how to extract style from one image and apply the style to another image (e.g. neural style transfer) or how to train a neural network to generate images. When we think about how these exciting research can be applied to astronomy, it can help us to understand more about our Galaxy. We have demonstrated we can do real science with this data generated by neural networks, and have real science impact instead of just a hype.
What do you hope will be your next step, professionally?
I have more than two years until my expected graduation date, so I still have some time to think about my future. Currently I am still not sure if I will be staying in academia or going to industry to work but no matter which path I choose, astronomy will always be part of my life as work or hobby.
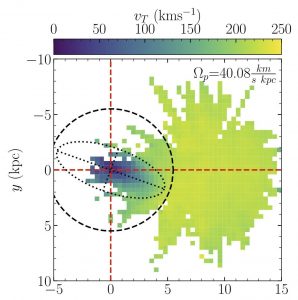
A plot showing the tangential velocity of Milky Way disc stars with a galactic bar (centred at the origin where our Sun at (8, 0)) in the centre with spectro-photometric distances from our neural network model. Credit: Henry Leung.
_________________________________________________________________________________________